Table of contents
Introduction
On a daily basis, we are confronted with outdated beliefs on the supposedly predestined roles of women in our society. Beyond these stereotypes, women are also confronted with specific beauty standards, such as being thin, having long blond hair, being young or having smooth skin. Notably, these beauty standards are reflected in the movie industry, with famous actresses such as Marilyn Monroe, Brigitte Bardot, Grace Kelly, and more contemporary figures like Margot Robbie, embodying them.
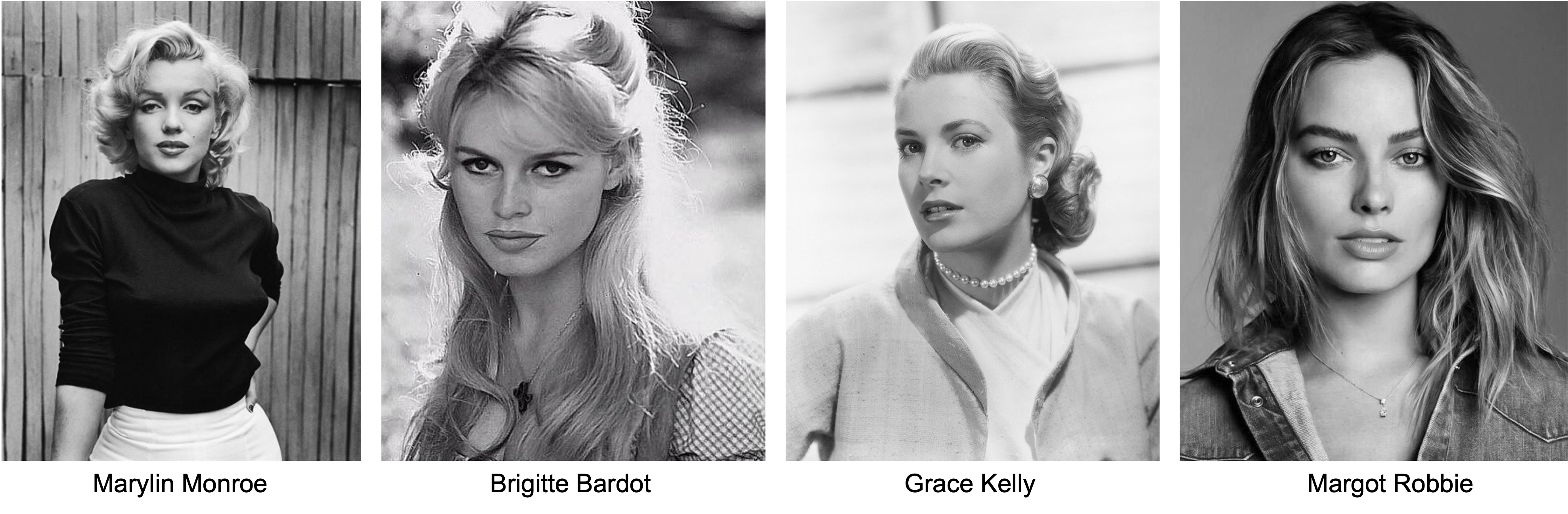
An interesting question to ask is whether stereotypes about women's roles also manifest in the movie industry, similar to how beauty standards do. What types of characters do women portray, and how often do they occupy main roles compared to men?
Additionally, diverse cultural backgrounds in various countries can shape the representation of women differently, and we can for example compare Hollywood, Bollywood, and European cinema. One might anticipate, for instance, that Hollywood places a strong emphasis on masculinity in its blockbuster productions. European films may lean towards more artistic and intellectual narratives, potentially fostering greater gender equality. Meanwhile, Bollywood's focus on family-centric themes could impact the roles and portrayal of actresses in the industry.
In this analysis, we want to determine whether and to what extent stereotypes persist in this industry. How are women represented in movies ? What type of characters do they interpret ? Can the representation of women have an impact on a movie's success ?
Dataset used
For this analysis, we used mainly the CMU Movie Summary Corpus, which contains data about 42'306 movies and about their characters. Besides, we used the supplement of this dataset consisting of the movie summaries parsed using the Stanford CoreNLP pipeline.
We also used three additional datasets to complete the analysis: The Movies Dataset, which contains information on a movies's cast in order to extract the main character and its associated gender. IMDb ratings, which is an online database of information about movies, TV series and more, where users can rate the movies they watch on a scale of 1 to 10. We used it mainly for IMDb's average rating and the number of votes per movie. The Oscar Award Dataset, which lists the movies that have been nominated and rewarded by the Academy Awards.
Note: most graphs are interactive, allowing you to hover over them, zoom in or out and explore further details.
Part 1: Quantifying gender inequalities
First, we need to explore quantitatively the extent of inequalities in the representation of women: are actresses really younger than actors? What is the difference between the proportion of actresses and actors? Which movie genres are more prone to inequalities?
Age of actors and actresses
Let’s look at the age of actors and actresses! The age is a first easy insight into our data, and at the same time quite symptomatic of gender stereotypes. As expected actresses are usually younger than actors:
T-test Distribution of Age between Actors and Actresses
P-value | Statistic | |
---|---|---|
T-test | 0.0 | -138.8 |
And the observed difference is significantly different with a nearly null p-value. We are not surprised by this information as this is the first impression we get when looking at most popular movies. Older men and younger women in relationships are the common trope of many movies. This is symptomatic of societal gender norms, women are at the peak of their beauty and career when they are young, while men age like good wine.
Distribution of female and male characters
Another interesting first insight is to examine the representation of men and women on screen, meaning looking at the distribution of female and male characters in the movies from our dataset. Let’s plot the proportion of female characters:
We observe a very skewed distribution! Indeed, many films have no female characters at all. How can this be? We can't be sure that no women appear in the movie, but they don't appear in the list of characters, which means that they are not among the most seen characters. What's more, the same is not true for men: none of the films have no male characters at all! We see a clear inequality in the representation of men and women on screen.
Does this representation change with movie genres ? We want to see if some genres are more equal, at least in terms of the proportion of characters. Let’s plot this distribution for the 16 most represented genres in our data set:
We observe that the movie genre with the lowest percentage of female characters is 'short film,' closely followed by 'action' and 'adventure,' as well as 'crime fiction', with a percentage of female characters lower than 30%. Among the 16 most represented genres, the one with the highest percentage of female characters is the 'Romance' genre. This illustrates the perception that men are often portrayed as adventurous and courageous heroes, while women are predominantly defined by their romantic relationships.
Part 2: Gender roles in movies
Now that we have quantified gender inequalities in the movie industry, we want to know if it has consequences on character representation: can we find typical roles per gender, and if so, what are they?
First a quick introduction in this theme with the type of character dataset. Are female roles in movies aligned with societal stereotypes? Or is it the representation of women on screen that also influences our society? We won't be able to answer this question, but we can start by visualizing common character tropes by gender:
World Cloud
Not surprisingly, the main characters the researchers were able to isolate are highly stereotyped! Actresses are portrayed as ‘dumb’ and the focus is only on their beauty, whereas men are characterized more by their actions (‘corporate’ , ‘hero’, …).
Characters clustering
To go deeper in this analysis we want to cluster the characters ourselves starting from the plots of the movies. The authors of the dataset used the CoreNLP toolkit to parse the movie plot summaries. We then extract the meaningful information about the characters: the action of which they are the agent or patient, and their attributes. With all of that information, we cluster the characters to identify similar ones:
To get a better idea of what character are contained within the clusters, we show them colored as a function of the proportion of female characters within each of them:
The cluster analysis revealed that a large majority of clusters contain a proportion of women similar to the one in the original dataset; thus these clusters are not particularly gendered. However, some clusters have a percentage of female characters significantly different from the baseline percentage. Clusters 7, 13, 34, and 15 contain fewer than 20% female characters, while Clusters 11, 17, and 28 exceed a 45% female representation. We visualize how the character are described in those gendered clusters with wordclouds:
Clusters with fewer women emphasize agent verbs associated with control or aggression (e.g., assure, arrest, plan, destroy, attack, warn) and patient verbs impacting behavior (e.g., interrupt, flirt, punch, punish, destroy, trap, or execute). In contrast, clusters with more women use agent verbs linked to emotional expression (e.g. love, remember, mention, unite, hope, greet, announce, promise) and patient verbs exploring emotions (e.g., disappoint, urge, communicate, chastise, nurture, bear, welcome, anger). Attributes in these clusters reveal additional contrasts: fewer women clusters feature attributes related to authority, opposition or high-level professions (e.g., poison, rebellious, prison, political, psychologist, banker, ambassador), while more women clusters highlight emotional or familial attributes (e.g., absent, calm, widow, fiancée, lovely, grandson, nephew, maid, babysitter). These differences in verbs and attributes potentially reflect underlying gender biases or societal norms embedded in character portrayal and storytelling. Clusters with fewer women might emphasize action-oriented narratives, authority, or conflict-driven storylines. Conversely, clusters with higher female representation might prioritize emotional depth or familial connections, emphasizing relationships and character development within storytelling contexts. However, it is important to highlight that the majority of the clusters don’t show such marked gender differences.
Classification of characters by gender
It's interesting to cluster character types together, but let's do a little quiz to see if, by knowing the words that characterize a character, we can find out its gender! If we are able to find it, this means that the roles are very stereotypical.
Quiz: Can you guess the character gender ?
You have classified words according to your preconcieved ideas on gendered roles! This suggests that it is usually possible to classify stereotipical characters from their attributes and actions. Let’s see if a machine learning classifier could do it! We provide the classifier with the agent verbs, patient verbs and character attributes as input features, and it outputs the predicted gender.
Classifier Results
Accuracy | Loss | |
---|---|---|
4 layer Simple Neural Network | 0.658 | 0.633 |
An accuracy rate of 0.66 suggests that the classifier has a certain level of predictive power above randomness. This indicates that there might be a discernible bias in the roles typically portrayed by women and men in movies: first, women might frequently be cast in nurturing, supportive, or romantic roles, whereas men might often portray heroic, dominant, or action-oriented characters. Second, female characters might sometimes lack depth or complexity compared to their male counterparts, being relegated to secondary roles or one-dimensional stereotypes. Finally, gender biases might influence how emotions are depicted, with women often shown as more emotional or sensitive, while men are portrayed as stoic or aggressive.
Sentiment analysis
Another interesting insight on the movies we can get through the plots is its general sentiment. Is the movie plot rather positive, neutral or negative ? And concerning our topic of interest, does the proportion of female characters or on the gender of the main character influence the sentiment of the movie? To perform a causal analysis, we matched the movies on the release year, the movie genre, and the country of release.
Linear Regression Sentiment ~ Main Character Female
Coef | P-value | |
---|---|---|
Intercept | 0.0416 | 0.000 |
Main Character Female | 0.0118 | 0.000 |
The coefficient associated with the percentage of females to predict the sentiment of the movie is significant but very low. Thus the percentage of females is slightly positively associated with the sentiment of the movie. However, as the Bechdel test highlights, it is not sufficient to have women in a movie: the Bechdel test is passed only if: (1) the movie has at least two women in it, who (2) who talk to each other, about (3) something besides a man. To dig deeper in this, let’s analyze the influence of the main character’s gender on the sentiment.
Linear Regression Sentiment ~ Percentage of Female
Coef | P-value | |
---|---|---|
Intercept | 0.0296 | 0.000 |
Percentage of Female | 0.0004 | 0.000 |
Movies with a feminine main character tend to have a more positive sentiment than movies with a masculine main character as the coefficient representing a feminine main character is positive, thus increasing the sentiment score. Indeed, the portrayal of female characters holding a substantial role and their experiences can influence the sentiment of a movie, shaping it towards themes like empathy, nurturing, resilience, and emotional depth. This could lead to a more nuanced and emotionally resonant storytelling style.
Part 3: Public perception of gendered movies
Now that we have understood the differences in women's representation in the movie industry itself, we are interested in the consequences they have on the public! It seems that blockbusters often feature men as their main characters. When examining the 10 movies with the highest box-office revenue of all time on the Wikipedia page List of highest-grossing films, we see that none of these movies showcase a female character as the sole main character, in contrast to several movies where a male character takes on the role of the sole main character.
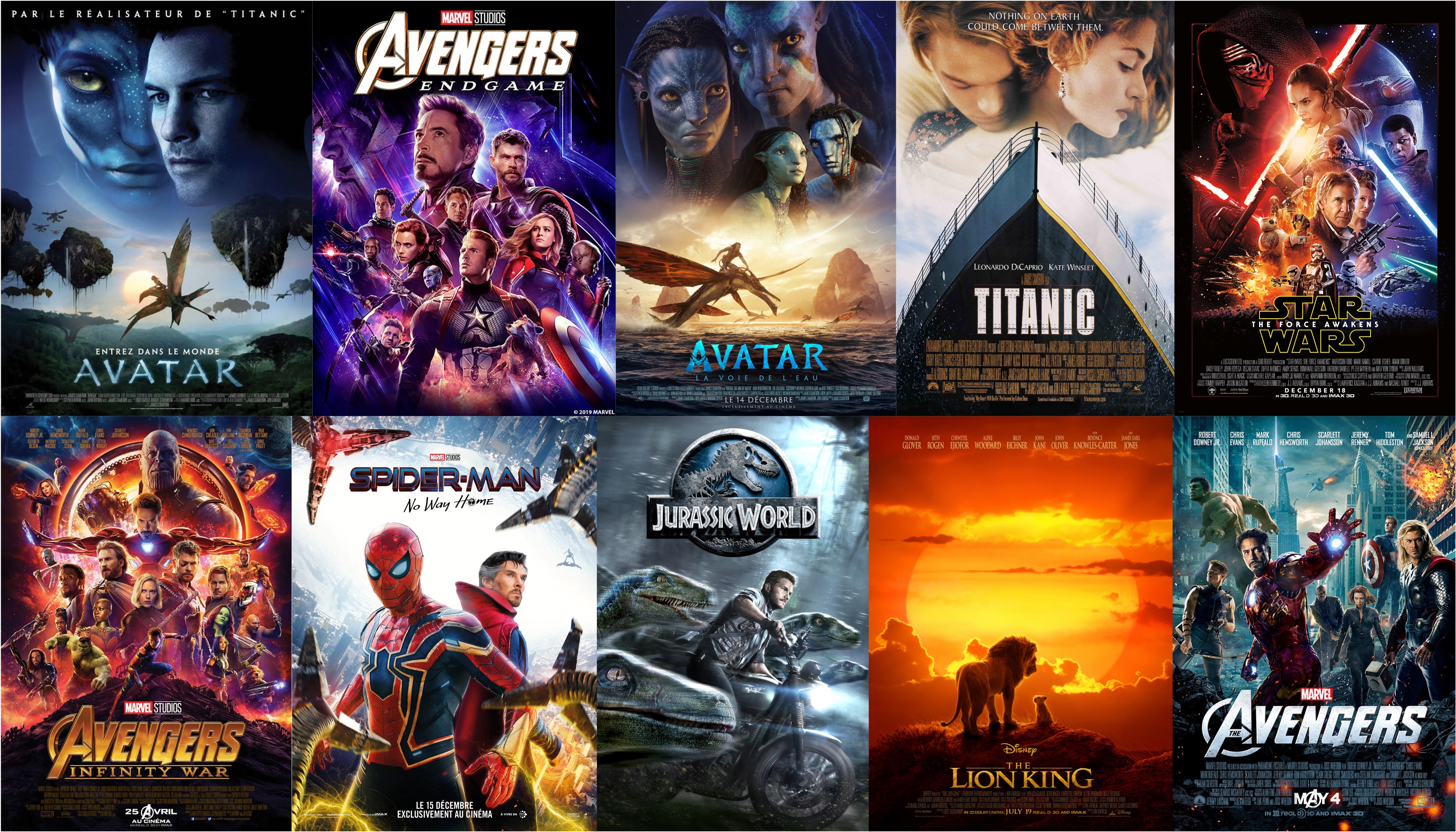
Perception of the public
First, we conducted an observational study to examine how the proportion of female characters in a movie correlates with its rating. We initially divided the dataset into two distinct groups: movies with a percentage of female characters above the median (treatment group) and those below the median (control group). Subsequently, to enhance the study's robustness, we executed exact matching based on release year (binned into 5-year intervals), country, and movie genre. Following this, we applied linear regression analysis, obtaining the subsequent results:
Linear Regression AverageRating ~ Percentage of Female Characters
Coef | P-value | |
---|---|---|
Intercept | 6.2102 | 0.000 |
PercentageofFemale | -0.0019 | 0.000 |
To conclude this first causal analysis, we see that the percentage of female characters has a significant negative impact on the movie rating at the 95% significance level, as the p-value is smaller than 0.05, and the coefficient is slightly negative. Thus the rating given by IMDB users is slightly decreasing with an increasing percentage of women in the movie.
The main character of a movie is also very important: indeed, the portrayal of the main character influences how audiences perceive the movie's themes, messages, and overall quality. That’s why we performed a second observational study, but this time on the gender of the main character's influence on the movie rating. Like in the previous causal analysis, we performed matching, where the treatment group consists of movies for which the main character is a woman, and the control group consists of movies for which the main character is a man. We then performed a linear regression to see how the main character influences the movie rating.
Linear Regression: Average Rating ~ Feminine Main Character
Coef | P-value | |
---|---|---|
Intercept | 6.3579 | 0.000 |
MainCharFemale | -0.0769 | 0.000 |
The presence of a slightly negative coefficient, coupled with a p-value below 0.05, suggests that movies featuring a woman as the main character tend to receive lower ratings from IMDb users compared to those with a man as the main character.One hypothesis is that viewers might have preconceived ideas about the type of roles women should play in movies. Therefore, when female characters challenge or deviate from these expectations, it can lead to discomfort or dissatisfaction among some audience members, affecting their perception of the film. But even though we conducted a causal analysis, a bias might be introduced by the audience diversity of IMDB or by the success of the director of the movies.
Perception of the film industry
The Oscars are one of the most prestigious and globally recognized awards in the entertainment industry, specifically for the film sector. Organized by the Academy of Motion Picture Arts and Sciences, the Oscars honor excellence in various categories related to filmmaking. Thus, to explore the potential influence of the main character's gender on a movie's reception within the film industry, we sought to examine its correlation with the likelihood of receiving an Oscar nomination.
We first excluded gendered Oscar categories for actors and actresses, which would bias our results; we also excluded categories that do not seem to be related to the main character, and so neither to its gender. Then we conducted a new causal analysis by matching exactly movies on their release-year period, their genre and their production country. Then we performed logit regression to predict if a movie was likely to be nominated for the Oscars based on the gender of its main character.
Coef | P-value | |
---|---|---|
Intercept | -2.0854 | 0.000 |
MainCharFemale | 0.1103 | 0.171 |
Our logit regression analysis revealed that the gender of the main character does not emerge as a statistically significant factor in predicting a movie's likelihood of receiving an Oscar nomination. Despite our exploration into this aspect, our findings suggest that the portrayal of the main character's gender may not really influence the movie's recognition by the Oscars.
While an Oscar nomination holds significant weight as a recognition of a film's excellence, it's essential to recognize that it represents only one facet of the film industry's reception. The assessment of a movie's success and reception within the industry is multifaceted, encompassing various critical reviews, festival recognitions, and other awards.
Part 4: Inequalities around the world
Now that we have analyzed the general inequalities that women face in the movie industry and the impact of gender on a movie's success, we will explore whether the cultural background of a country can alter this impact, since stereotypes about women vary from culture to culture. To do so, we will examine how genders are represented across three geographical areas—USA, India, and Europe—each representing significant movie industries: Hollywood, Bollywood and European movies.
Our aim is to analyze the percentage of women in movies in the 3 different regions of interest: USA, India and Europe. To do this, we can study the following map, which represents the average percentage of female characters in films by country, worldwide.
If we compare the different regions we're interested in, we can see that India has one of the highest percentages of women in movies, at almost 35%. The United States is a little behind with a percentage of women of around 30%. In Europe, on the other hand, we can see a segmentation between Eastern and Western Europe, where the percentage is much higher in the West (around 30%) than in the East (around 20%). We calculated the average percentage of female characters in movies from each of these three regions, and obtained the following results: for the USA, the average is 33.7%, for India, 33.5%, and for Europe, 31.6%. There seems to be only a slight difference, but let’s take a closer look at these numbers!
Differences in gender representations between European, Indian and American movies
First, to address potential confounding variables that might influence the gender representation between the USA, Europe and India, we performed exact matching based on the release year and the movie genre. As a result, the following analysis is done on triplets of movies from the 3 regions of interest that have the same release year and genre. However, exact matching based solely on release year and movie genre might not account for all potential confounders or nuances influencing gender representation in films. Factors like cultural context, directorial choices, scriptwriting, and societal norms could still impact the portrayal of women in movies. Then, after performing the matching, to compare the average percentage of women between the 3 regions of interest, we performed an ANOVA, and obtained the following results:
ANOVA: Mean percentage of female characters of Indian, American and European movies.
Statistic | P-value | |
---|---|---|
ANOVA | 14.31 | 6.23e-07 |
As the p-value is smaller than 0.05, this implies that the difference in the percentage of female characters is significantly different in at least one of the three regions. However, it does not tell us which groups are different from each other. We therefore carried out a post hoc test, the Tukey test, to make pairwise comparisons between the means of each group. We found that the difference between the percentage of actresses was significantly different between the EU and the US, and between the EU and India. The results of this test are visualized on the boxplot below:
Distribution of the percentage of female characters in Indian, American and European movies
The test results reveal an unexpected trend: European movies tend to feature approximately 2% fewer feminine characters compared to American and Indian cinema. This finding challenges common perceptions, as European cinema might be stereotypically associated with a cultural inclination towards more balanced or prominent portrayals of feminine characters, distinct from American or Indian cinema.
Conclusion
So, what did we find in our analysis? First we showed that there is a quantitative difference in the way actors and actresses are treated on screen. We also showed that the role distribution is gendered, meaning some character tropes are more feminine and others masculine. Then we looked at how the public and the Oscar Academy perceived those different types of movies. Finally we focused our attention on finding the differences between diverse cultural backgrounds that shape the representation of women. The results were not as expected and we learned that European movies tend to have less actresses on screen. We learned that we have to be careful with our preconceived biases !